Previously, retailers made decisions about what items to sell, where to sell them, how much stock to keep, and when to change prices based on their experience and intuition. While many retailers are proud of their instincts, relying on them alone is not enough anymore, especially with tight profit margins. Consumer preferences change quickly, and retailers can’t track all market factors accurately.
Data analytics software can help retailers make better and more profitable decisions by enhancing or correcting their educated guesses. Want to know more about it? This beginner’s guide will show businesses everything they need to know about retail analytics and its best practices.
Table of Contents
Retail analytics: what is it, and what are its benefits for businesses?
The 4 types of retail data analytics
5 best practices to help businesses get the most out of retail analytics
Types of tools retailers can use for retail analytics
Final words
Retail analytics: what is it, and what are its benefits for businesses?
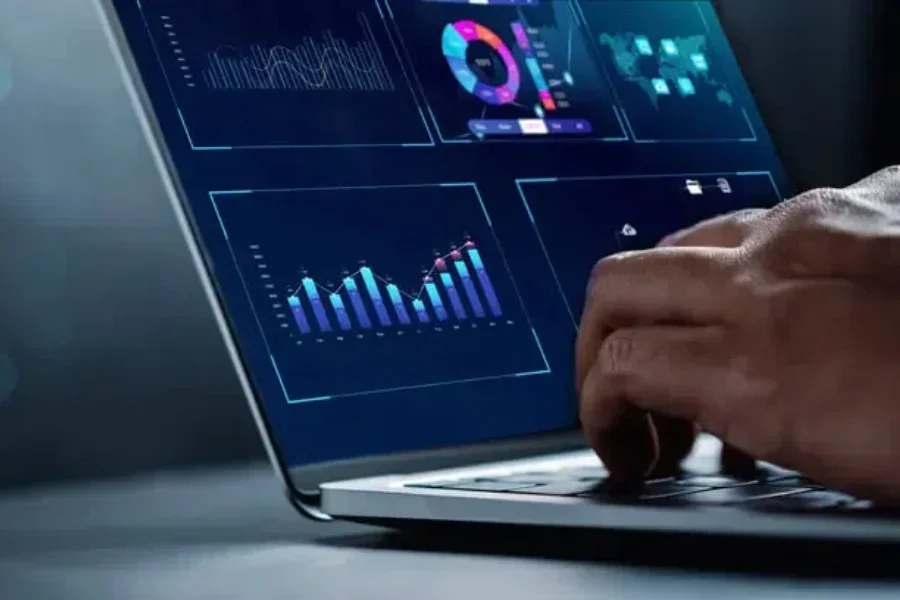
Retail analytics uses software to gather and analyze data from physical stores, online shops, and catalogs to help retailers understand customer behavior and shopping trends. This information can improve decisions on pricing, inventory, marketing, product placement, and store operations by using predictive algorithms on data from both inside the business (like customer purchase histories) and outside sources (like weather forecasts).
Retail analytics can also measure customer loyalty, spot buying patterns, forecast demand, and enhance store layouts. For example, it helps retailers place frequently bought-together items on shelves and offer personalized discounts to regular customers, leading to bigger purchases and more visits.
Benefits of using retail analytics for business
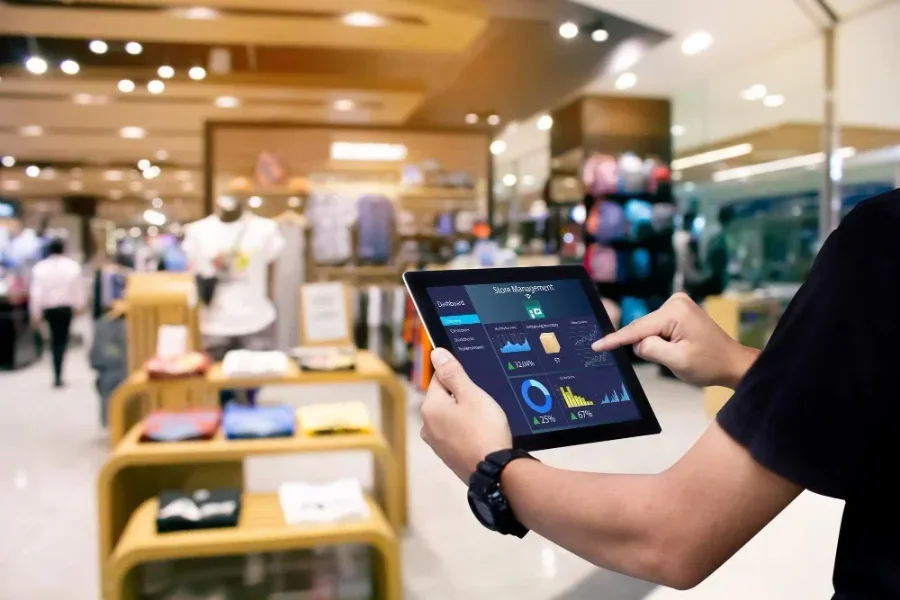
Reducing stockouts and discounts
By understanding demand trends, retailers can stock the right amount of products. For example, analytics can show how quickly demand drops for consumer electronics influenced by social media, helping retailers avoid overstocking and heavy discounting.
Enhancing personalization
Analytics help retailers understand what their customers like, allowing them to capture more sales. For instance, a book retailer can use purchase history to notify customers interested in American history about a new book by historian Ron Chernow available for preorder.
Optimizing pricing decisions
Data analytics can help retailers set the best prices by considering factors like abandoned shopping carts, competitor prices, and product costs. This ensures prices are neither too high nor too low, maximizing profits.
Improving product allocation
Analytics can guide retailers on where to send products, reducing unnecessary transport costs. For instance, a retailer for sports clothing can leverage analytics to see that even a slight temperature drop increases thermal undershirt sales, so they can send more to colder areas.
The 4 types of retail data analytics
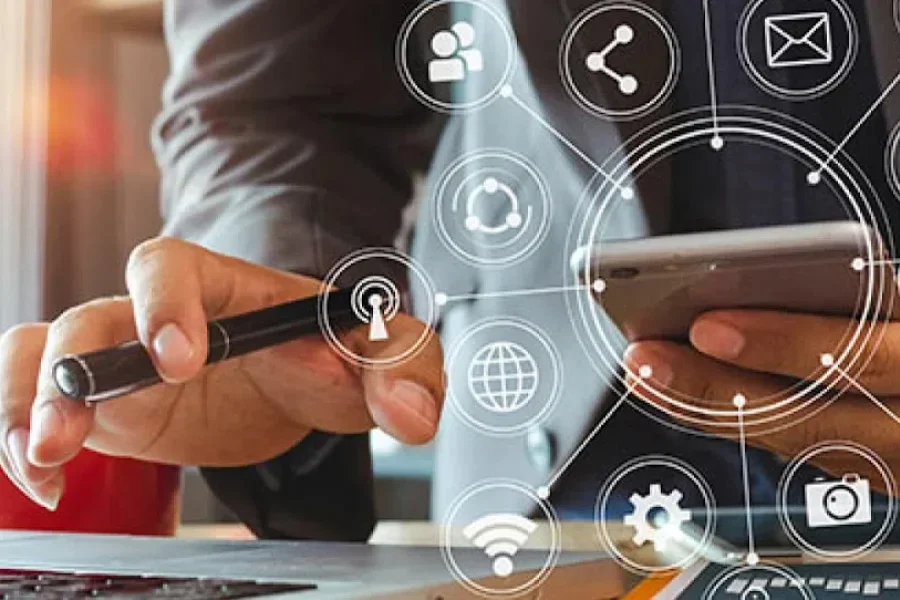
Descriptive analytics
Descriptive analytics looks at past performance to answer basic questions like “how many,” “when,” “where,” and “what.” It’s the basis of business intelligence tools and dashboards that show frequent reports on sales and inventory.
Diagnostic analytics
Diagnostic analytics helps find the causes of problems affecting performance. Combining data from various sources, like customer feedback, financial results, and operational metrics, gives retailers a deeper understanding of their issues.
Predictive analytics
Predictive analytics forecasts future events by analyzing the weather, economic trends, supply chain issues, and competition. It often involves “what-if” scenarios, like predicting how effective offering a 10% discount will be over a 15% discount or estimating when stock will run out under different conditions.
Prescriptive analytics
Prescriptive analytics uses AI and big data to recommend actions based on predicted outcomes. For example, it can suggest offers for customer service agents, such as upselling based on purchase history or cross-selling to address new customer inquiries.
5 best practices to help businesses get the most out of retail analytics
1. Rely on customer data
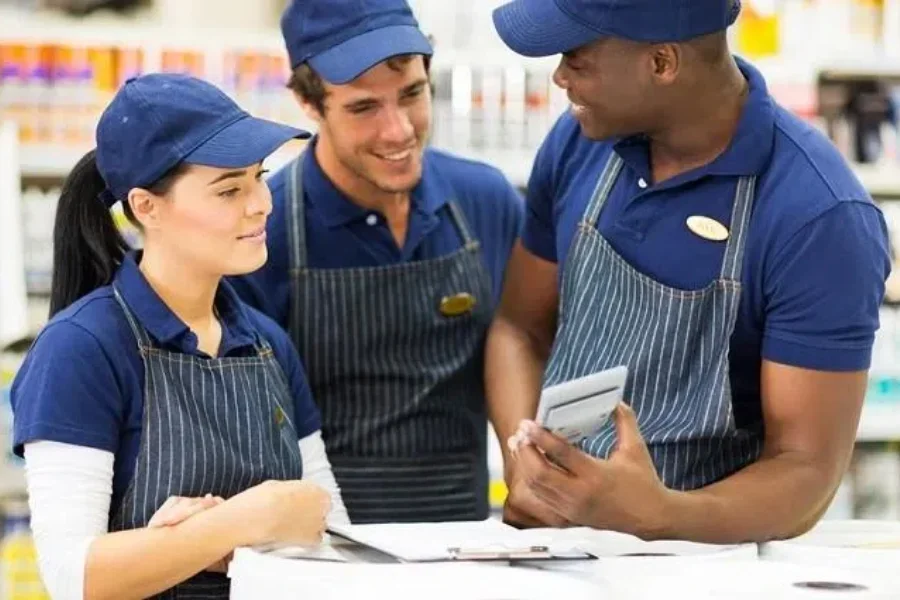
Customers share a lot of information about what they want and need. The best retailers use this data to spot trends and better understand their customers. They combine data from loyalty programs, e-commerce, POS systems, and other sources, including data purchased from brokers.
Usually, experts divide customer data into demographic, transactional, behavioral, and psychographic information. Retailers start by gathering basic demographic data and then expand to other types. They distinguish between “customers” (people who have bought from them) and “consumers” (potential customers). Consumer data helps in “lookalike modeling”—for example, if Mark is a great customer, the retailer looks for more people like Mark and targets them with special offers.
2. Use visualization tools
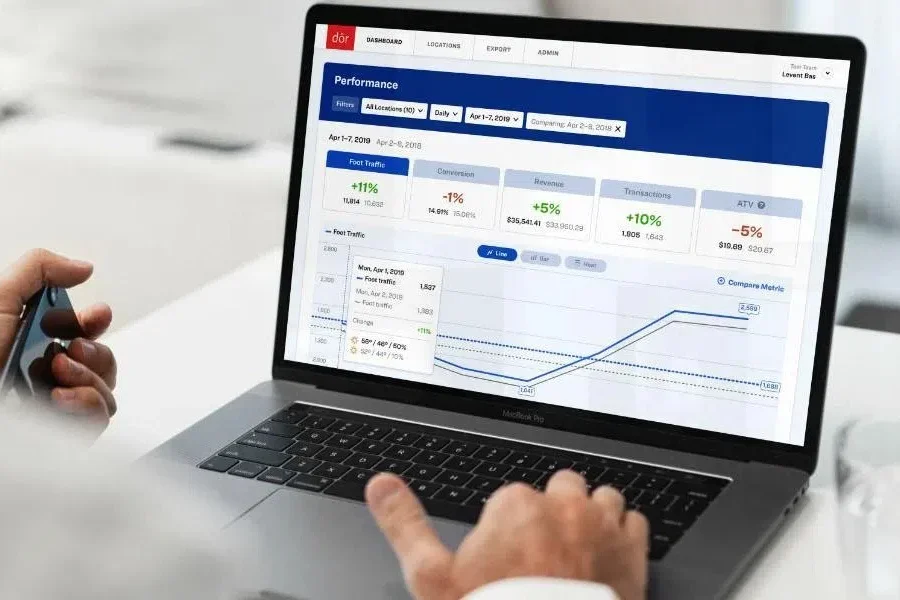
Charts, graphs, and dashboards, common elements in BI software, are crucial for understanding data and making smart decisions. They are much more effective than just looking at rows and columns of data. These tools also allow business users to access analytics directly without waiting for IT to create reports and run queries.
3. Look at different data sources
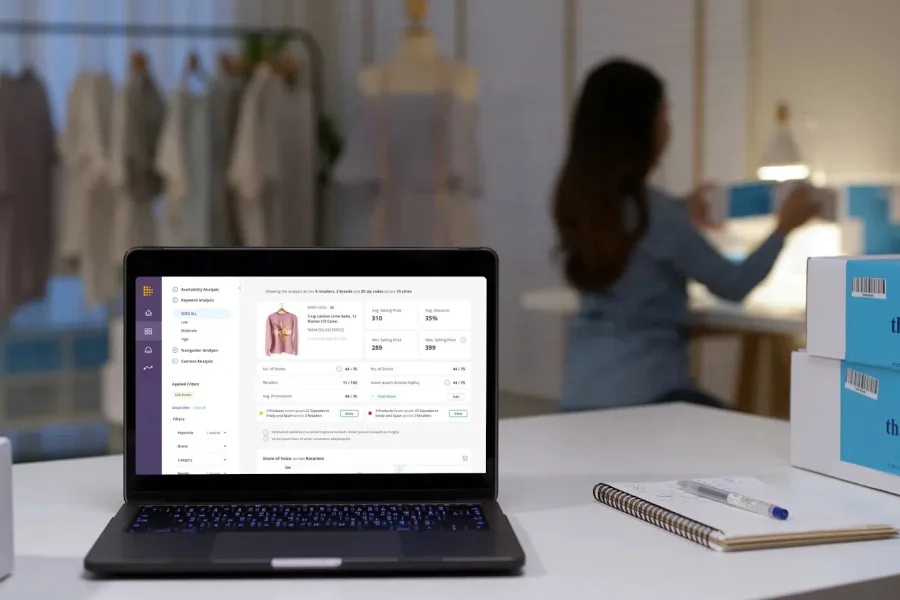
Looking at different data sources, like sales numbers, old customer data, and stock levels, helps stores understand their business better. Why? These numbers are often connected in some way. For example, stores can link in-store data with product details to determine the best store layout to convert visitors into buyers.
Checking stock levels ensures there are enough products to match the store layout. Stores should also keep in mind that different tools may define data in various ways, which can lead to mistakes if not fixed. This is a good reason to use one platform for all retail data instead of picking the best tool for each job.
4. Track important numbers
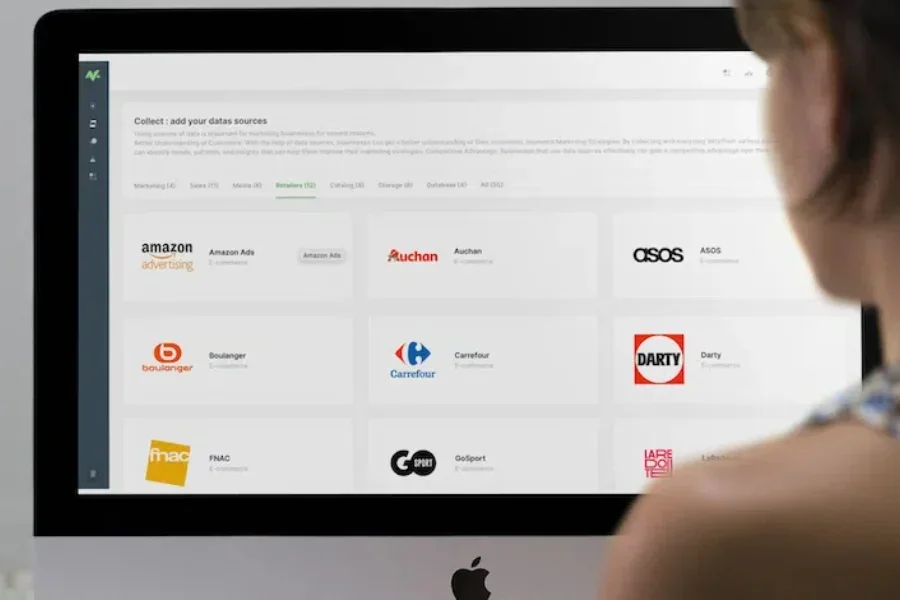
Watching important numbers helps stores see how well they are doing and find ways to improve. The most successful stores look at these numbers every week, comparing them to the week before. This starts with looking at what happened (like certain items selling less) and then figuring out why it happened (like running out of stock).
5. Focus on main goals
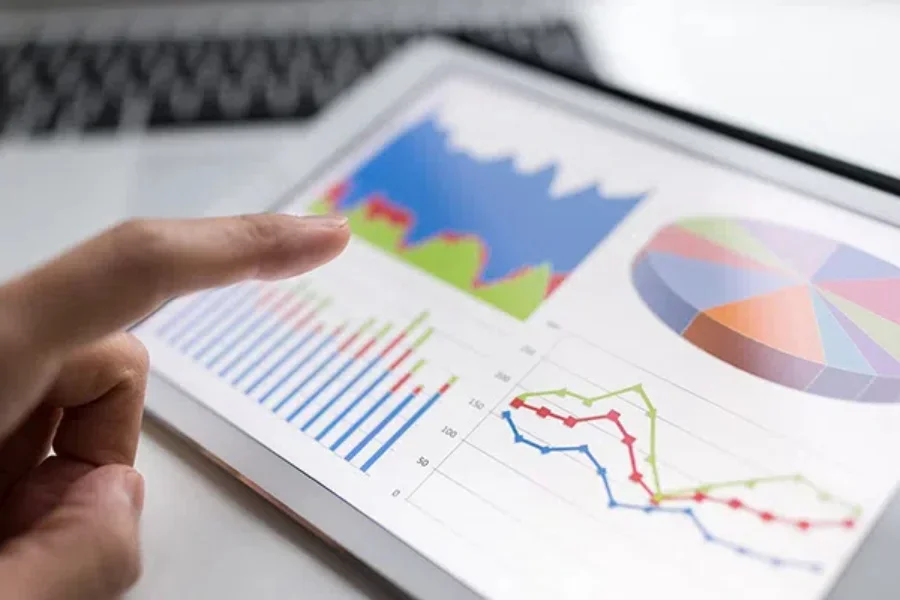
Not everything should be measured. Retailers have many new tools and data, but they must choose wisely or risk overwhelming decision-makers. Instead, they should first find key areas that can quickly improve the business. According to McKinsey, the best analytics solve a specific problem and lead to clear results.
Types of tools retailers can use for retail analytics
Retail analytics uses data collected from stores and websites through various tools, including the following:
1. Point-of-sale (POS) systems
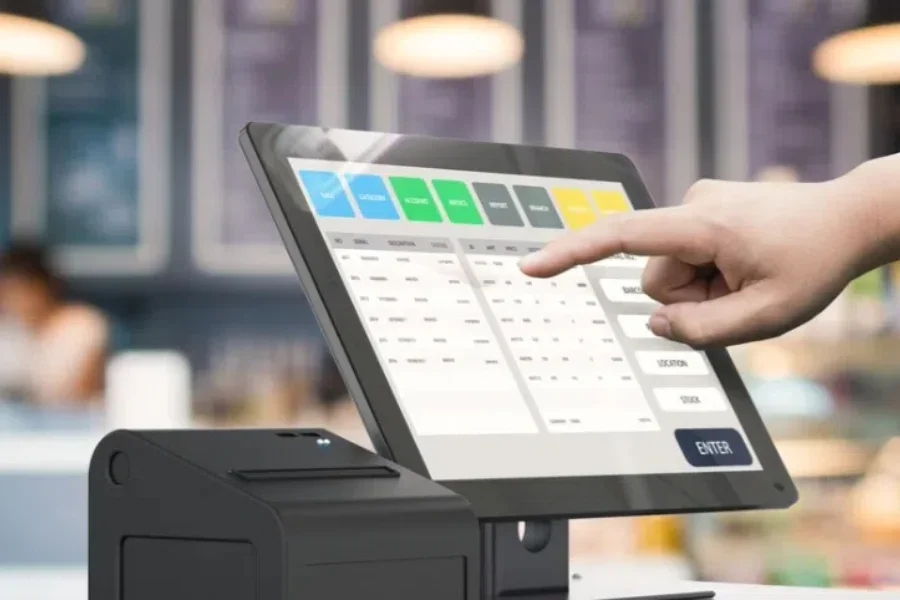
These systems track and manage customer transactions. They provide data on what customers buy and can create reports on sales and trends.
2. Customer relationship management (CRM) software
This software manages sales, marketing, customer service, and online processes. Retailers use it to track customer interactions, keep information about customers, and find new sales and marketing opportunities.
3. Business intelligence (BI) tools
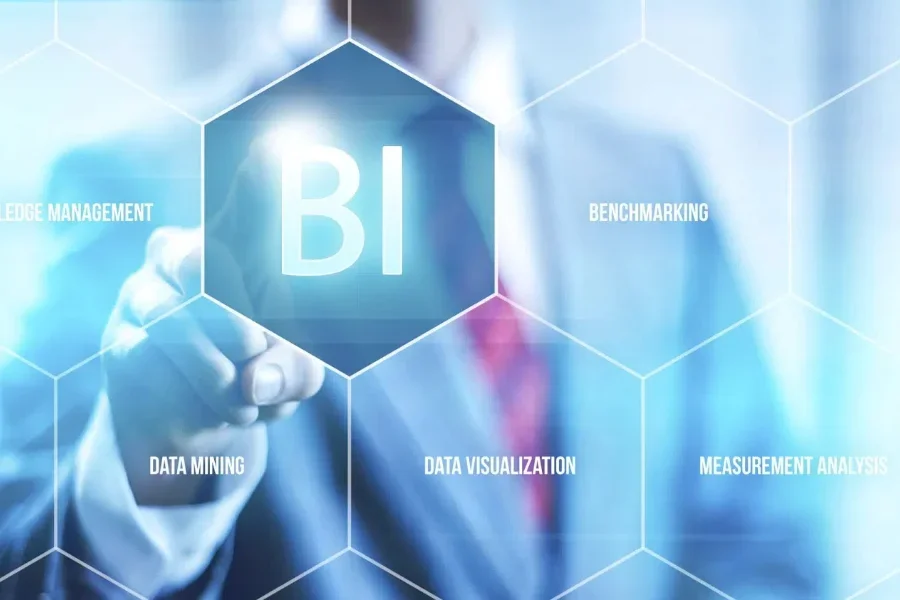
BI tools combine data from different sources to track key performance indicators like customer loyalty, inventory turnover, and sales rates. They can generate reports for executives and other decision-makers.
4. Inventory management systems
This software tracks stock levels in stores and warehouses, forecasts demand, and helps decide where to store items to reduce costs and meet customer needs.
5. Predictive analytics
This type of analytics uses past data to forecast future trends and behaviors. The main types of retail analytics are descriptive, diagnostic, predictive, and prescriptive. These help identify growth opportunities and new customer groups.
Final words
Retail analytics are an essential part of any business making sales. It helps keep track of the business and make future predictions to avoid stock-outs and other detrimental situations. While relying on intuition was the norm, retail analytics is slowly (but surely) phasing it out. Data in 2024 is not the same as 20 or 10 years ago, so retailers must evolve if they want to handle all incoming and outgoing data.