Every year-end, alongside the joyous holiday season and hopes for the new year, another “entertainment” that now seems to be a tradition rooted in modern New Year celebrations is predictions for the forthcoming year. From psychics to industrial experts, people are enthralled by all such forecasts, eager to glean about the impending future.
Similarly, in a world saturated with data now, Statistical Forecasting—the scientific method of analyzing historical data to predict future trends across various fields, be it finance and economics or supply chain and logistics—is often the linchpin in predicting the industrial future. However, with the advancement of Machine Learning, things are becoming more intriguing nowadays as it infuses both vibrancy and depth into Statistical Forecasting.
Continue reading to discover the connections between Machine Learning and Statistical Forecasting, the applications of Machine Learning in Statistical Forecasting today, and how Machine Learning can enhance Statistical Forecasting.
Table of Contents
1. Introduction to Machine Learning and Statistical forecasting
2. Key Machine Learning applications in Statistical Forecasting
3. How Machine Learning improves Statistical Forecasting
4. Precise predictions within reach
Introduction to Machine Learning and Statistical Forecasting
Brief overview of Machine Learning
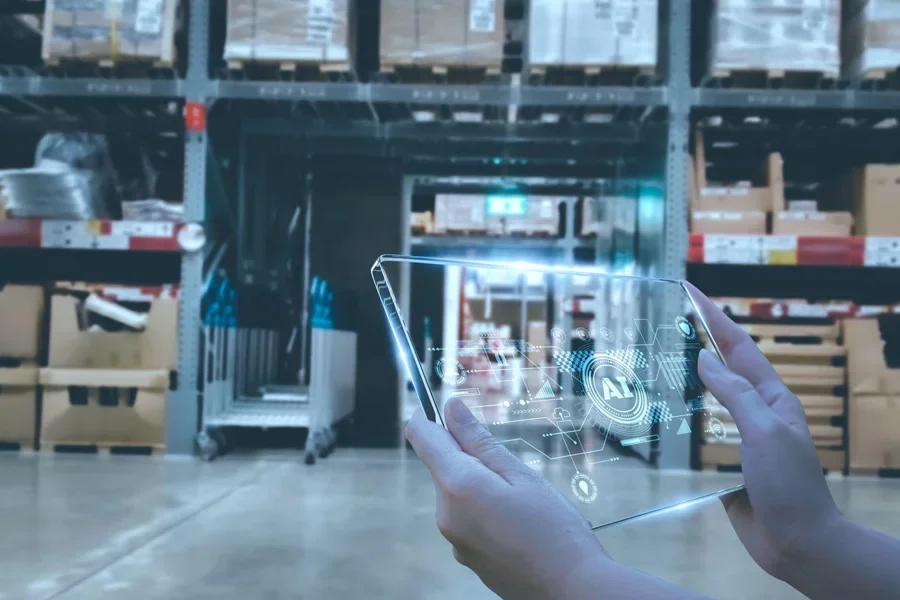
Machine learning, a subset of artificial intelligence and computer science, enables AI to learn in a manner similar to human learning. By leveraging algorithms and data, machine learning continually enhances its accuracy. The ability to process and analyze large amounts of data, detect patterns, and make predictions are some of its most prominent characteristics. For instance, about half of the top 10 machine learning applications significant to both industry professionals and everyday users are related to predictions and forecasts, including traffic conditions, customer preferences, and stock movements.
In essence, machine learning represents a significant advancement in predictive technology as it can discern complex patterns and relationships in data that may not be detectable to human analysts. It is also capable of continuous improvement in predictions over time, refining its effectiveness as more data is processed.
The basics of Statistical Forecasting
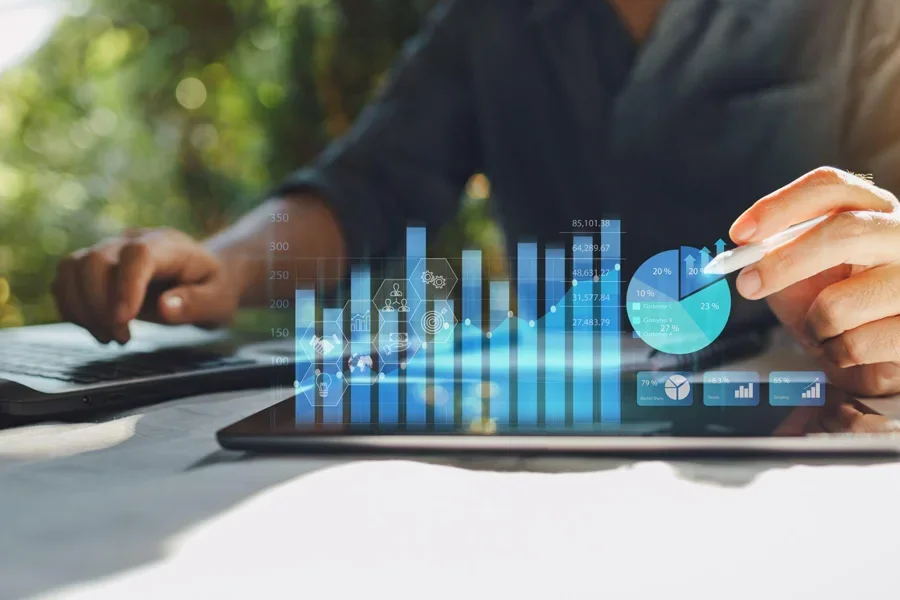
Statistical Forecasting utilizes historical data combined with statistical methodologies including sampling and hypothesis testing to identify trends, seasonal patterns, and correlations. These elements are used to model relationships and predict future outcomes. This method operates under the assumption that future patterns will reflect past ones.
Statistical methods such as time series analysis and regression models are prevalent and widely used across various fields. By analyzing past data, these models extrapolate and forecast future events with varying levels of accuracy, depending on the clarity and consistency of the data patterns.
In supply chain management, Statistical Forecasting is essential for demand planning, inventory management, and resource allocation, optimizing supply chain processes. Time series forecasting techniques such as ARIMA (Autoregressive Integrated Moving Average) and exponential smoothing are integral parts of this approach, in view of their robustness in trend analysis and adjustments for seasonality. Simply put, Statistical Forecasting serves as a cornerstone in the strategic planning and operational efficiency of supply chain management, leveraging time-tested methodologies to enhance predictability.
Machine Learning and Statistical Forecasting: Evolution and relationship
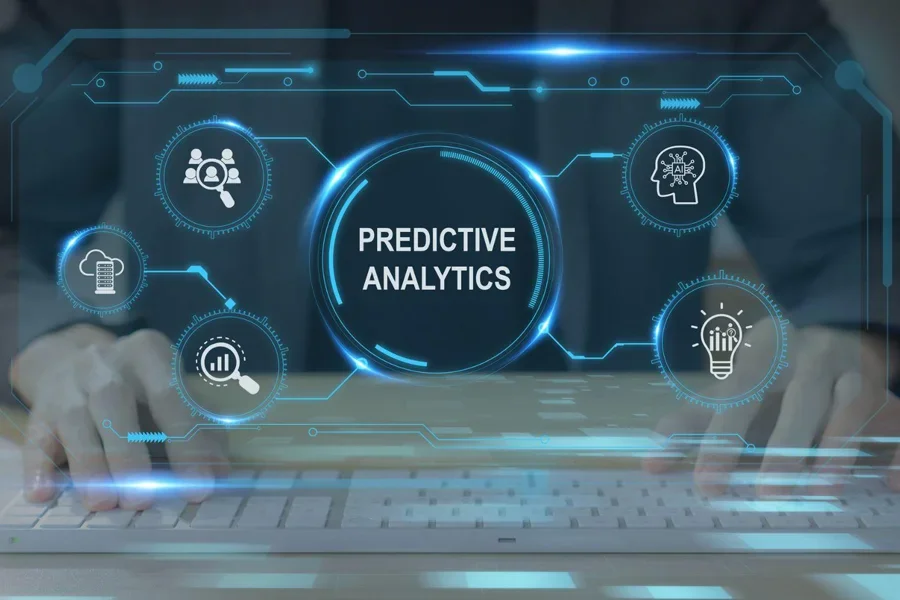
Modern supply chain management integrates increasingly sophisticated statistical and Machine Learning models to address the complexities of global logistics, diverse data sources, and real-time data flows. This shift from traditional statistical methods to Machine Learning-driven forecasting marks a significant evolution, highlighting the potential for supply chain management to adopt more dynamic, data-driven decision-making processes.
Initially, research and competition suggested that classical time series methods were superior to Machine Learning methods in forecasting accuracy. However, the 2020 M5 competition demonstrated the ability of Machine Learning, while building upon the foundations of classical time series forecasts, has caught up and even surpassed classical methods.
To date, Machine Learning is increasingly integrated with Statistical Forecasting and used alongside statistical methods to enhance overall efficiencies. This approach is not about replacing traditional methods outright; rather, it complements those methods by adaptively improving predictions over time, crucial for dynamic environments in the supply chain.
The evolution of forecasting has seen Machine Learning models become more prominent, challenging the dominance of traditional statistical methods like ARIMA and Exponential Smoothing. With their capabilities in processing and analyzing large datasets, uncovering complex patterns, and predicting trends, Machine Learning algorithms have offered significant enhancements in facilitating data-driven decisions, continuously refining forecasts.
Key Machine Learning applications in Statistical Forecasting
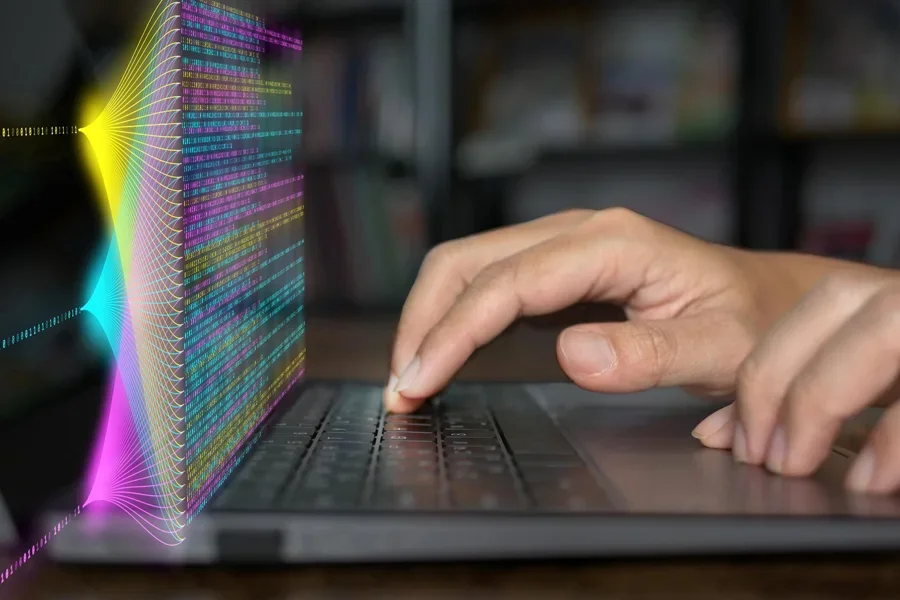
In supply chains, Machine Learning improves demand prediction through techniques like Demand Sensing, crucial for planning and optimizing logistics and inventory. This application improves inventory management by predicting consumer behavior and market trends, reducing overstock or stockouts, and enabling real-time trend analysis.
Machine learning is known for its superior ability to handle high-dimensional data without extensive preliminary model specification. It excels in processing diverse data, incorporating non-linearity and anomaly detection, thereby becoming invaluable for complex predictive tasks such as image recognition and fraud detection, where traditional statistical methods often struggle.
Essentially, Machine Learning is able to identify these complex patterns through learning phases conducted by neural networks, enhancing predictive capabilities beyond those of traditional models. By employing a variety of algorithms, Machine Learning offers enhanced data processing and adaptability to complex data environments. This enriches the capabilities of traditional forecasting methods and drives more data-driven decision-making.
How Machine Learning improves Statistical Forecasting
Enhanced forecasting accuracy
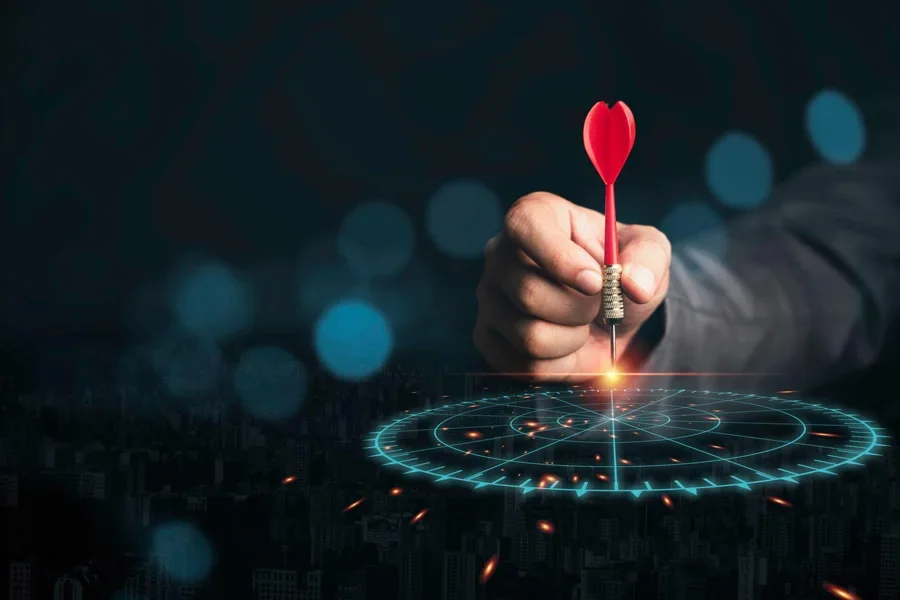
Machine Learning significantly enhances forecasting accuracy through its ability to process and analyze large and complex datasets, continually adapting to new data to refine predictions. In the supply chain, such forecasting accuracy directly impacts efficiency by reducing overstock and stockouts, ensuring better inventory management.
Additionally, it has been proven that Machine Learning models such as Random Forest, Light Gradient Boosting Machine (LightGBM), and eXtreme Gradient Boosting (XGBoost) enhance forecasting accuracy by aggregating predictions from multiple models. These models, utilized in ensemble methods, combine predictions from various simpler models, substantially improving forecasting precision. They achieve this by utilizing diverse data interpretations and reducing overfitting, thereby consistently surpassing traditional forecasting methods.
Furthermore, Machine Learning models like XGBoost and Long Short-Term Memory (LSTM) networks have shown superior performance over traditional statistical models by capturing complex patterns in data that significantly enhance forecasting accuracy. These Machine Learning models provide more accurate predictions by identifying complex patterns not typically discernible by traditional methods.
On the other hand, an implementation example demonstrates that a two-step classification process using decision trees and multitask neural networks enables Machine Learning to effectively determine the optimal statistical model and its parameters for specific SKU time series. This method shows how forecasting can use advanced learning strategies to smartly align the most suitable and unbiased statistical models with particular demand trends, notably enhancing how Machine Learning and traditional forecasting methods work together.
Granularity and adaptability
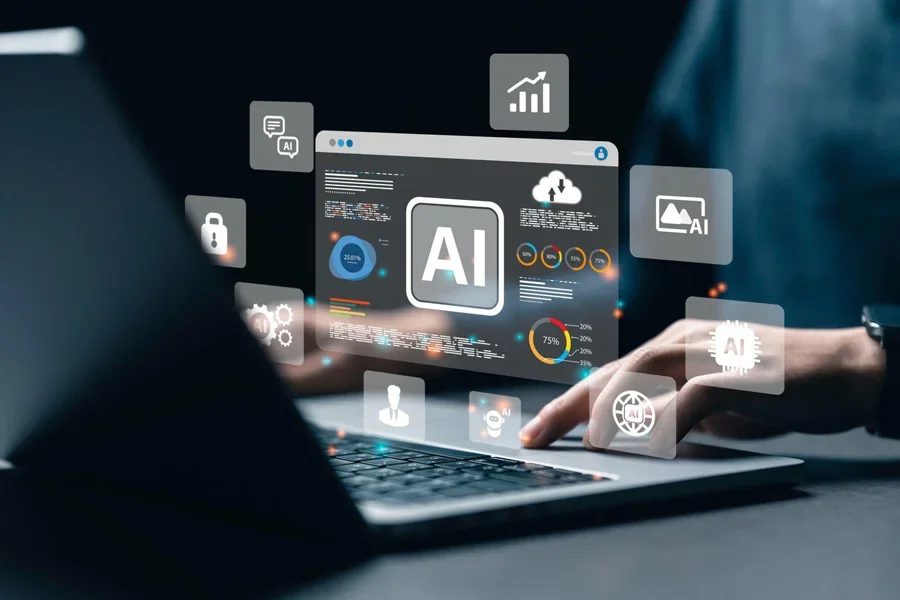
Statistical forecasting has inherently relied on its comprehensive approach to assessing and analyzing long-term historical data. As a result, it has typically been utilized for predictions in the long run rather than short-term fluctuations. Specifically, micro-forecasting has traditionally not been its strength. In contrast, the dynamic adaptability of Machine Learning models, particularly those employing online learning, allows them to rapidly adjust to new data.
This capability supports more granular and situation-specific predictions, adapting forecasts to real-time market conditions. Such flexibility is exemplified in Demand Sensing, enhancing the ability to provide more granular and adaptable forecasting, crucial for quick responses to supply chain disruptions or shifts in consumer demand.
Overall, Machine Learning enhances micro-forecasting in the supply chain by adapting to real-time changes. It effectively handles and adjusts to a vast array of complex, varied, and dynamic data patterns, further optimizing inventory and reducing waste. Consequently, Machine Learning stands as a pivotal tool in modern supply chain management, enabling businesses to react swiftly and efficiently to market changes and demand fluctuations.
Cost efficiency
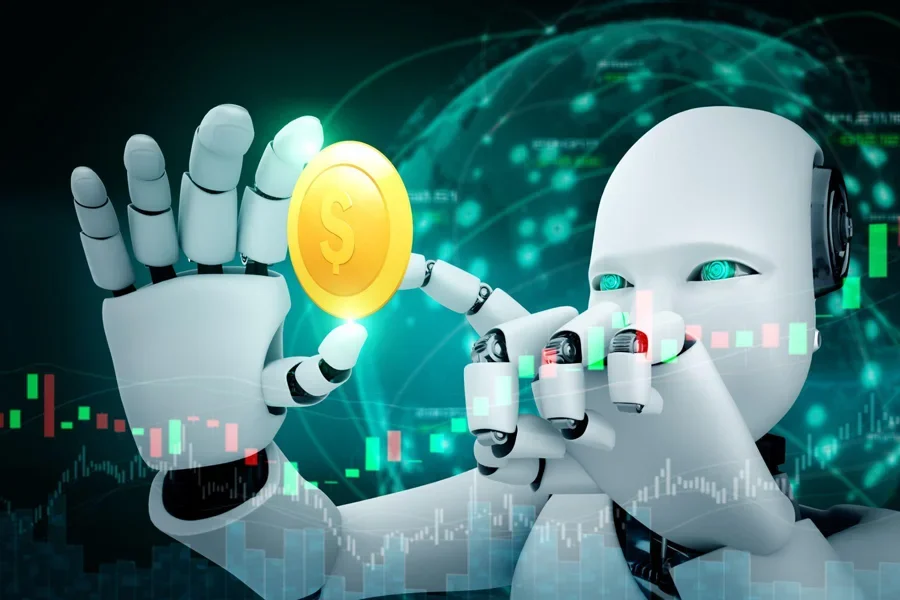
The impact of Machine Learning on enhancing cost efficiency in statistical forecasting is closely linked to its ability to improve accuracy. By automating data analysis processes, Machine Learning not only increases the precision of forecasts but also significantly reduces forecasting costs, which is crucial for large-scale supply chain operations.
The deployment of meta-learning, alternatively named “learning to learn fast”, a subset of Machine Learning, also enhances the performance of learning algorithms by making adjustments based on experimental outcomes. This approach helps to further decrease computational costs by minimizing the need for exhaustive searches across multiple forecasting models and parameters during each forecast cycle, thereby saving time and resources and remarkably enhancing cost efficiency.
Viewing from a broader perspective, Machine Learning also contributes to cost reductions related to excess inventory and lost sales. For instance, Machine Learning reduces costs associated with overstocking or understocking through more accurate demand forecasts. Moreover, through automated feature discovery, Machine Learning models also decrease the necessity for manual feature engineering, which can indirectly lead to further cost reductions in the model development phase.
Decision support
Machine Learning models, capable of quickly and accurately analyzing vast and complex datasets, provide robust decision support in dynamic environments. These models enhance decision-making processes where rapid and precise predictions are crucial, offering deeper insights and more reliable predictions. In supply chain management, specifically, such timely forecasting underscores the importance of better decision-making for procurement and distribution planning—crucial elements in managing logistics efficiently.
On top of that, Machine Learning equips decision-makers with forecasters and tools that swiftly identify the most effective forecasting methods, enhancing their understanding of demand patterns and forecast outcomes. This allows them to focus on refining forecasts where it matters most, optimizing the overall strategic planning process.
Finally, an essential and undeniable feature of Machine Learning in supporting decision-making is its ability to guarantee unbiased predictions based solely on data. This is a specific advantage of Machine Learning: it produces predictions that are purely data-driven, free from human biases, emotions, or subjective interpretations.
While Statistical Forecasting also relies on data and quantitative methods, the potential for human biases during model selection, parameter setting, and interpretation of results could influence the outcomes to some extent. Machine Learning, particularly with its automated and adaptive systems, mitigates this by processing large volumes of data continuously and adaptively in response to new information. As long as the data itself is free of bias, this approach can lead to more objective and unbiased predictions.
Precise predictions within reach
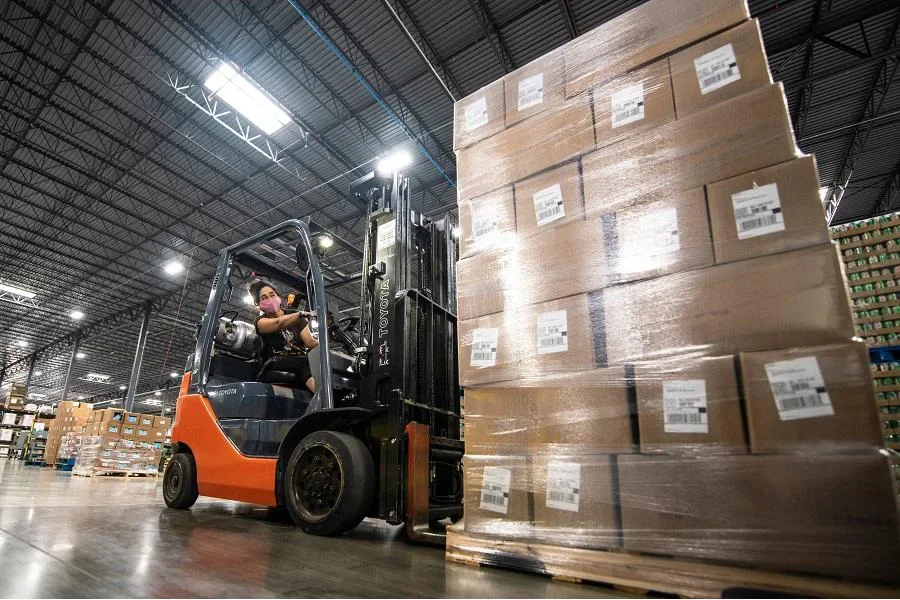
The integration of Machine Learning into statistical forecasting represents a significant advancement in predictive analytics. This combination enhances the accuracy of forecasts while also introducing an unprecedented level of adaptability and precision in handling diverse data sets and forecasting scenarios. Machine Learning algorithms contribute to creating precise predictions within reach by enabling more refined and contextually aware decision-making processes, both essential for the dynamic needs of modern businesses.
The benefits of Machine Learning extend beyond accuracy; they include improvements in cost efficiency and the capability to utilize comprehensive datasets effectively. These advancements facilitate a deeper understanding of market trends and consumer behaviors, thereby supporting more strategic decision-making across various sectors. Through Machine Learning, organizations are equipped to make more informed decisions, optimizing operations and reducing risks associated with poor forecasting. The future of forecasting is set to achieve even greater integration of these technologies, continually improving the scope and accuracy of predictive analytics.
Discover how Machine Learning is transforming forecasting across industries by regularly visiting Chovm.com Reads– an essential resource for wholesale business insights, news, and strategies that can make a difference.
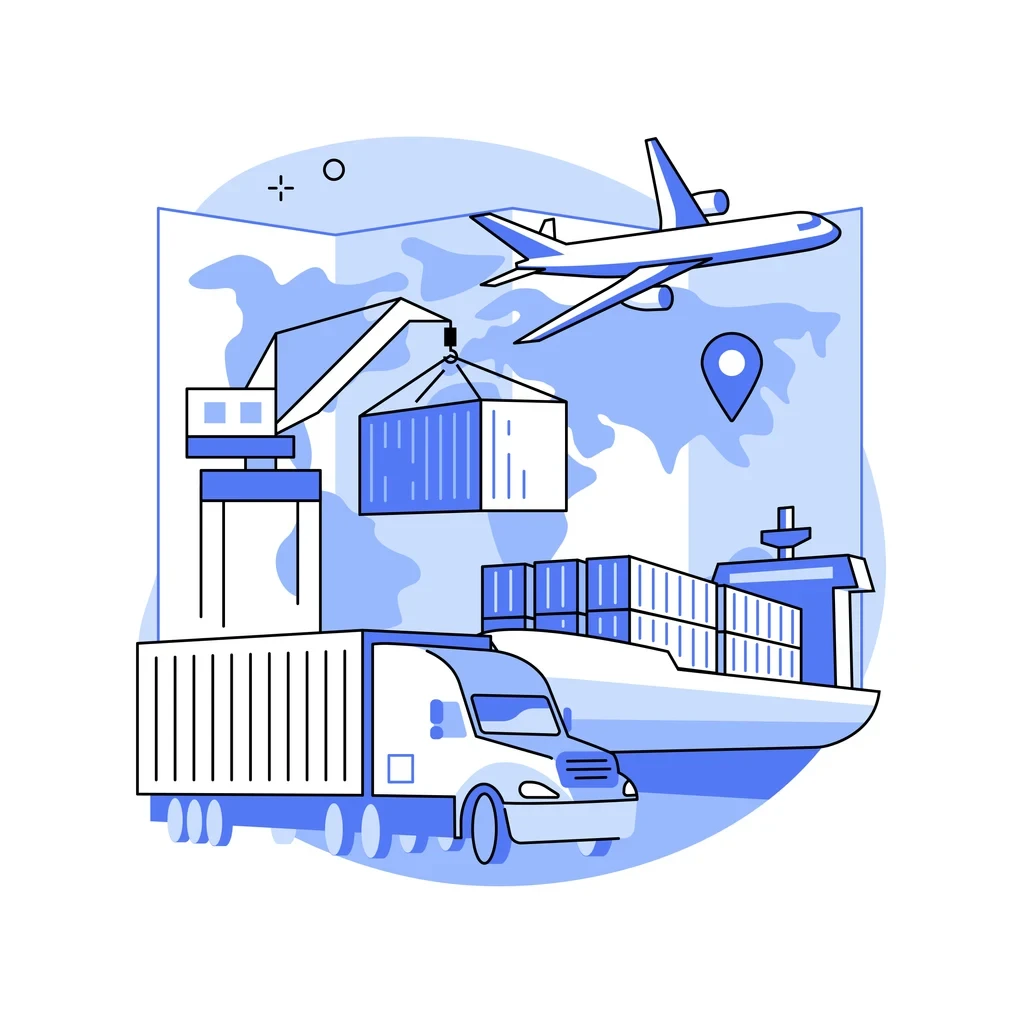
Looking for a logistics solution with competitive pricing, full visibility, and readily accessible customer support? Check out the Chovm.com Logistics Marketplace today.